ScholarSphere: IITJ Research Insights Hub
ScholarSphere: IITJ Research Insights Hub is to preserve and enable easy access to the Intellectual output of its faculty members, such as Journal Papers, Conference Papers, Books, Book Chapters, Reports and Preprints to the research community.
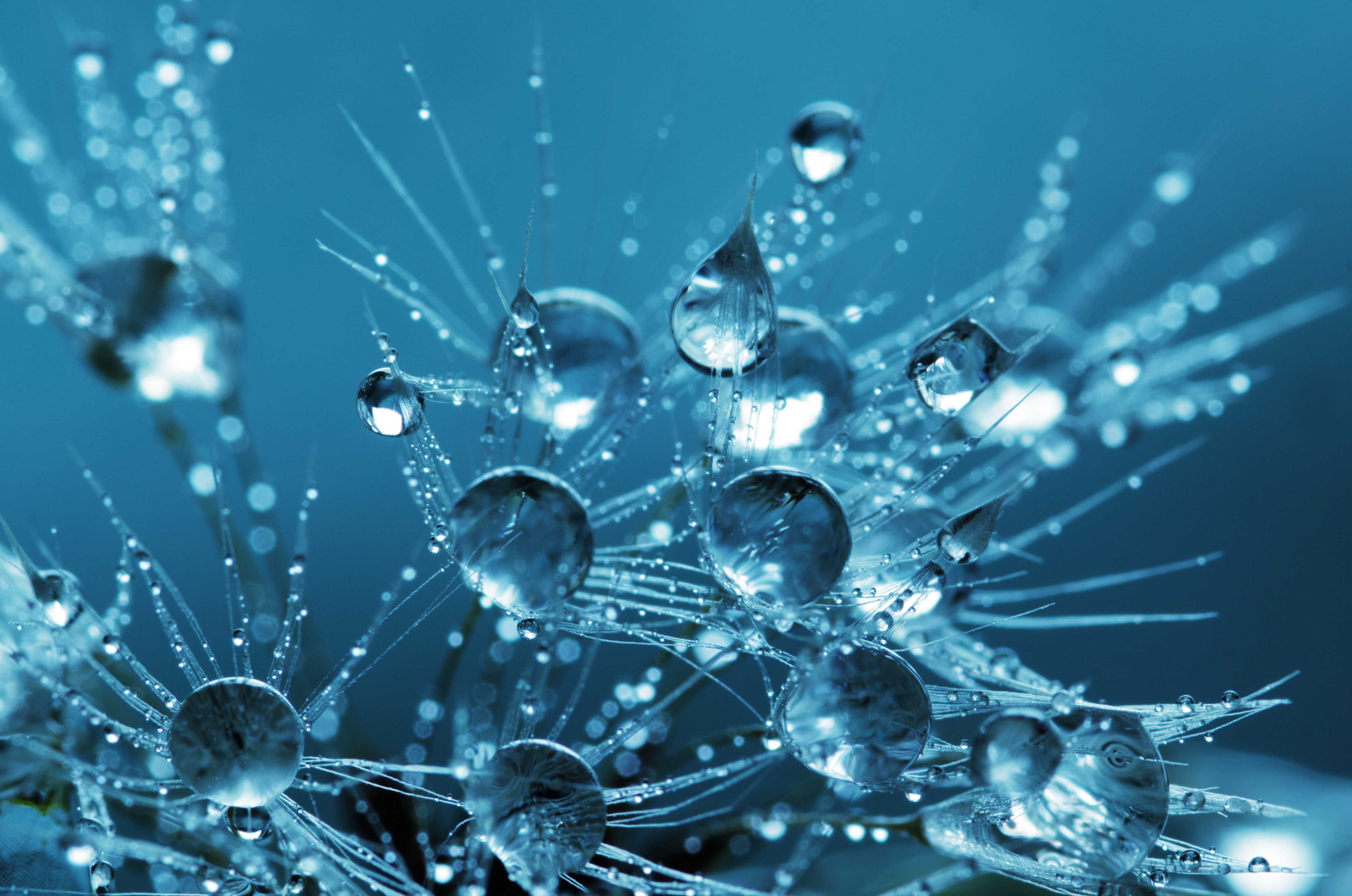
Research outputs
17
Projects
0
People
265
Recent Additions
- PublicationA novel predictor coefficient interpolation algorithm for enhancement of spatial resolution of images(2010)This paper presents a novel algorithm for enhancement of spatial resolution of images. The proposed algorithm estimates a Least square based predictor of lower order and interpolates the coefficients of higher order predictor. We have reduced the predictor order form p to (p-1) that results into a saving of computational power. The proposed algorithm is generic that can be used with most of the LS based interpolation algorithms reported in literature. We have shown that use of interpolated prediction coefficient causes insignificant loss in subjective as well as objective (PSNR) quality of the higher resolution (HR) image as compared with the PSNR obtained by the actual prediction coefficient and there is around 40% to 50% reduction in computational complexity.
- PublicationHigh Dimensional Neural Networks and Applications(2010)Intelligent systems are emerging computing systems developed based on intelligent techniques. These techniques take advantage of artificial neural networks to emulate intelligent behavior. Extensive studies carried out during the past several years have revealed that neural networks enjoy numerous practical advantages over conventional methods. They are more fault-tolerant, less sensitive to noise and mostly used for their human-like characteristics (learning and generalization). They have been accepted as powerful tools for correlating data without making strong assumptions about the problems. Traditional neural networks's parameters are usually real numbers for dealing with real-valued data. However, high-dimensional data also appear in practical applications and consequently, high-dimensional neural networks have been proposed. They have also presented improved results even in case of real-valued problems. As a prelude, we provide a brief overview of the existing methodologies in high-dimensional neural computation. Our particular point of view is to describe several real-world applications, in which the use of these techniques really helps in achieving the goals of intelligent system.
- PublicationFeedback fiber delay lines and AWG based optical packet switch architecture(2010)All-optical switching has been proposed as a candidate to allow high capacity networking in the future. However, most of the proposed all optical switching architectures have a large physical loss, and hence, their cascadabilty is limited. This paper proposes an optical packet switch (OPS) which has very low loss. The architecture is realized by using components like optical reflectors, tunable wavelength converters (TWCs), arrayed waveguide grating (AWG) and pieces of fiber. This architecture uses the routing pattern of AWG, and its symmetric nature, to simplify switch operation significantly. It is also shown that by using a multi-wavelengths optical reflector, the length of delay lines can be reduced to half of its original value. This reduction in length is useful for larger size packets as their length can grow to more than a few kilometers. The proposed architecture is compared with other approaches that have been published in literature in terms of performance, implementation complexity, number of components used and cost. This paper also proposes a cost model for the cost estimation of the TWCs. The proposed model takes into consideration fiber-to-chip coupling (FCC) and the wavelength speed-up factor (WSU).
- PublicationThe novel aggregation function-based neuron models in complex domain(2009)he computational power of a neuron lies in the spatial grouping of synapses belonging to any dendrite tree. Attempts to give a mathematical representation to the grouping process of synapses continue to be a fascinating field of work for researchers in the neural network community. In the literature, we generally find neuron models that comprise of summation, radial basis or product aggregation function, as basic unit of feed-forward multilayer neural network. All these models and their corresponding networks have their own merits and demerits. The MLP constructs global approximation to input-output mapping, while a RBF network, using exponentially decaying localized non-linearity, constructs local approximation to input-output mapping. In this paper, we propose two compensatory type novel aggregation functions for artificial neurons. They produce net potential as linear or non-linear composition of basic summation and radial basis operations over a set of input signals. The neuron models based on these aggregation functions ensure faster convergence, better training and prediction accuracy. The learning and generalization capabilities of these neurons have been tested over various classification and functional mapping problems. These neurons have also shown excellent generalization ability over the two-dimensional transformations.
- Person
Most viewed
- Person
- Person
- Person
- Organizational Unit
- Person